Mirror Mirror: Crowdsourcing Better Portraits
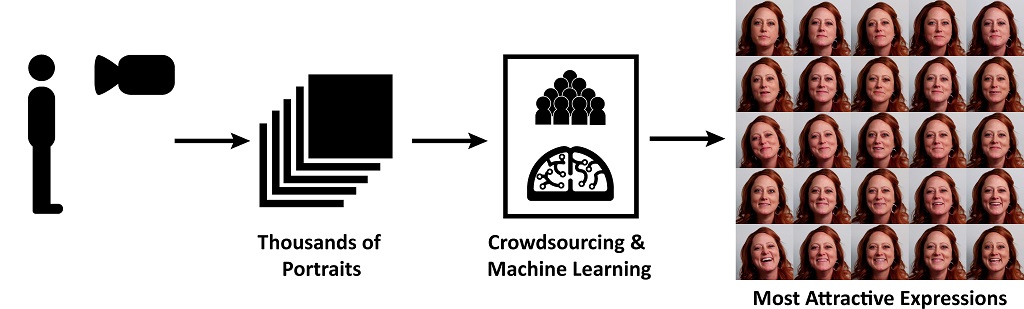
People
Video
Abstract
We describe a method for providing feedback on portrait expressions, and for selecting the most attractive expressions from large video/photo collections. We capture a video of a subject’s face while they are engaged in a task designed to elicit a range of positive emotions. We then use crowdsourcing to score the captured expressions for their attractiveness. We use these scores to train a model that can automatically predict attractiveness of different expressions of a given person. We also train a cross-subject model that evaluates portrait attractiveness of novel subjects and show how it can be used to automatically mine attractive photos from personal photo collections. Furthermore, we show how, with a little bit ($5-worth) of extra crowdsourcing, we can substantially improve the cross-subject model by “fine-tuning” it to a new individual using active learning. Finally, we demonstrate a training app that helps people learn how to mimic their best expressions.
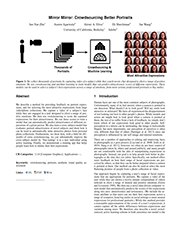
Paper
SIGGRAPH Asia paper. (pdf, 48MB)
Reduced-size SIGGRAPH Asia paper. (pdf, 2.6MB)
Presentation
(pptx + videos), 136MBCitation
Jun-Yan Zhu, Aseem Agarwala, Alexei A. Efros, Eli Shechtman and Jue Wang. Mirror Mirror: Crowdsourcing Better Portraits.
ACM Transactions on Graphics (SIGGRAPH Asia 2014). December 2014, vol. 33, No. 6.
Bibtex
Additional Materials
- Supplemental Material, 43MB
Include additional attractive/serious ranking results and visualization results.
Software
-
MirrorMirror: An expression training App that helps users mimic their own expressions.
-
SelectGoodFace: This program can select attractive/serious portraits from a personal photo collection. Given the photo collection of the *same* person as input, our program computes the attractiveness/seriousness scores on all the faces. The scores are predicted by the SVM models pre-trained on the face data that we collected for our paper. See Section 8 and Figure 17 for details.
-
FaceDemo: A simple 3D face alignment and warping demo.
Data
Data (898MB) include the original videos, the selected representative frames, attractiveness/seriousness scores estimated from crowdsourced annotation, and extracted HOG features. We also provided MATLAB code to visualize scores and train cross-subject svm models.
Acknowledgement
We thank Peter O’Donovan for code, Andrew Gallagher for public data, and our subjects for volunteering to be recorded. Figure 1 uses icons by Parmelyn, Dan Hetteix, and Murali Krishna from The Noun Project. The YouTube frames (Figure 16) are courtesy Joshua Michael Shelton.Funding
This research is supported in part by:
- Adobe Research Grant
- ONR MURI N000141010934