Learning a Discriminative Model for the Perception of Realism in Composite Images
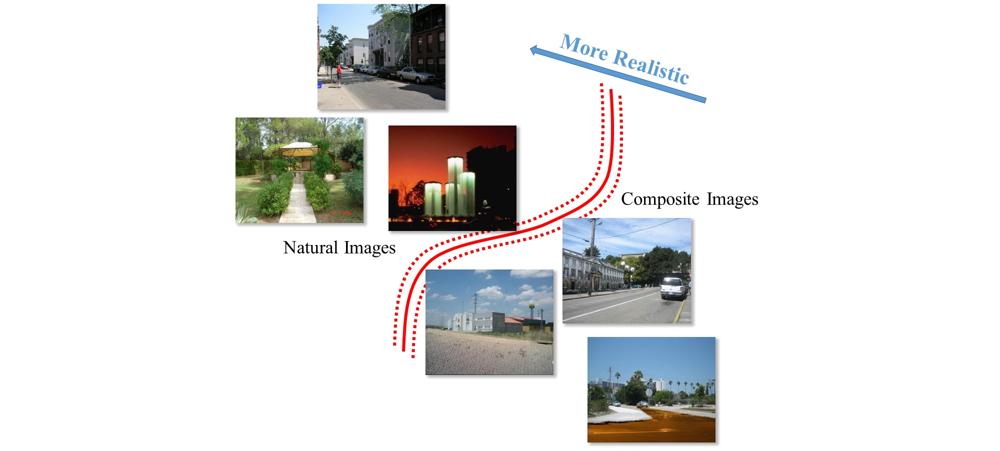
People
Abstract
What makes an image appear realistic? In this work, we are answering this question from a data-driven perspective by learning the perception of visual realism directly from large amounts of data. In particular, we train a Convolutional Neural Network (CNN) model that distinguishes natural photographs from automatically generated composite images. The model learns to predict visual realism of a scene in terms of color, lighting and texture compatibility, without any human annotations pertaining to it. Our model outperforms previous works that rely on hand-crafted heuristics, for the task of classifying realistic vs. unrealistic photos. Furthermore, we apply our learned model to compute optimal parameters of a composition method, to maximize the visual realism score predicted by our CNN model. We demonstrate its advantage against existing methods via a human perception study.
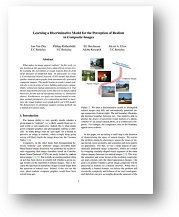
Paper
ICCV 2015 paper, 2.6MB
Presentation
pptx, 16MBPoster
ICCV poster, 1.8 MBCitation
Jun-Yan Zhu, Philipp Krähenbühl, Eli Shechtman and Alexei A. Efros. "Learning a Discriminative Model for the Perception of Realism in Composite Images", in IEEE International Conference on Computer Vision (ICCV). 2015. Bibtex
Code
-
RealismCNN: implements (1) realism prediciton and (2) color adjustment methods described in the paper. To run the code, please also download the models and data.
Data
-
RealismCNN Models: caffe models and test prototxt. We include both models trained on different LabelMe datasets (15 largest object categories vs. all the object categories). We also include models with/without hard negative mining.
-
Realism Prediction Data: Test data for comparing different realism prediction methods.
-
Color Adjustment Data: Test data for comparing different color adjustment methods.
-
CNN Training data: photographs used for CNN training (natural photos vs. image composites).
LabelMe_15_object_categories includes ~ 11, 000 natural images containing ~ 25, 000 object instances from the largest 15 categories of objects in the LabelMe dataset. Most of these images are outdoor natural scenes.
LabelMe_all_object_categories includes ~ 21, 000 natural images (both indoor and outdoor) that contain ~ 42, 000 object instances from more than 200 categories of objects in the LabelMe dataset. -
Caffe prototxt for training
Additional Materials
- Supplemental Material: This document includes the gradient derivation of Equation (1) and (2) in the main paper.
- Photo Realism Ranking: This includes the ranking of all 719 photos according to our model's visual realism prediction. The color of image border encodes the human annotation: green: realistic photos; red: unrealistic composites; blue: natural photos.
- Color Adjustment: This includes the color adjustment results for all 719 images in the dataset from Lalonde and Efros [15]. Each rows shows the object mask, cut-and-paste, Lalonde and Efros [15], Xue et al. [33] and our result. Below each result, we show the human rating score estimated from pairwise comparison annotations. Please check Section 6.1 in the main paper for detailed discussion.
Acknowledgement
We thank Jean-François Lalonde and Xue Su for help with running their code.
Funding
This research is supported in part by:
- ONR MURI N000141010934
- Adobe research grant
- Intel research grant
- NVIDIA hardware dontation
- Facebook Graduate Fellowship